Using hypervolumes in your own algorithmΒΆ
In this tutorial we will show how to use the hypervolume features in your own custom evolutionary algorithms. If you haven’t already, we suggest getting familiar with the tutorial Adding a new algorithm.
As it is often the case in the tutorials, we will present a fairly simple MOO algorithm, which we would not expect to be particularly good.
from PyGMO import *
from PyGMO.util import *
import random
class my_hv_moo_alg(algorithm.base):
"""
A custom steady-state algorithm, based on the hypervolume computation.
"""
def __init__(self, gen = 10, p_m = 0.02):
"""
Constructs an instance of the algorithm.
USAGE: my_hv_moo_alg(gen=10, p_m=0.02)
NOTE: Evolves the population using the least contributor feature.
* gen: number of generations
* p_m: probability of mutation
"""
# We start by calling the base constructor
super(my_hv_moo_alg,self).__init__()
# Store the number of generations
self.__gen = gen
self.__p_m = p_m
# Performs a very simple crossover step
def cross(self, ind1, ind2):
x1 = ind1.cur_x
x2 = ind2.cur_x
return tuple(random.choice((x1[i], x2[i],)) for i in xrange(len(x1)))
# Gaussian mutation
def mutate(self, x, lb, ub):
# Implementation of the Gaussian operator
def _g_op(i):
return min(max(random.gauss(x[i], (ub[i]-lb[i]) * 0.1), lb[i]), ub[i])
# Condition for the mutation to happen
def _rnd_mut():
return random.random() < self.__p_m
return tuple(_g_op(i) if _rnd_mut() else x[i] for i in xrange(len(x)))
# Evolve method
def evolve(self, pop):
#If the population is empty (i.e. no individuals) nothing happens
if len(pop) == 0:
return pop
#The algorithm now starts manipulating the population
prob = pop.problem
lb, ub = prob.lb, prob.ub
for s in range(self.__gen):
# Initiate new individual by a crossover of two random individuals
idx1 = random.randint(0, len(pop) - 1)
idx2 = (idx1 + random.randint(0, len(pop) - 2)) % len(pop)
ind1 = pop[idx1]
ind2 = pop[idx2]
new_x = self.mutate(self.cross(ind1, ind2), lb, ub)
pop.push_back(new_x)
# Remove the least contributor
hv = hypervolume(pop)
ref_point = hv.get_nadir_point(1.0)
lc_idx = hv.least_contributor(ref_point)
pop.erase(lc_idx)
return pop
def get_name(self):
return "Custom HV-based MOO"
def main():
prob = problem.dtlz(2)
alg = my_hv_moo_alg(gen = 100, p_m=0.02)
pop = population(prob, 100)
# Establish a constant reference point, so the increase is noticed
ref_point = (3000,) * 3
for _ in xrange(100):
pop = alg.evolve(pop)
print "P-Distance: %.5f, Hypervolume: %.5f" % (prob.p_distance(pop), hypervolume(pop).compute(ref_point))
prob.plot(pop)
if __name__ == "__main__":
main()
You can copy the whole code above and save it as a python script (e.g. my_alg.py), this way you can execute it yourself by issuing the following in the command line: python my_alg.py.
The algorithm does the following in the evolve method:
- Establish a new individual by performing a very simple crossover on two random individuals
- Apply the Gaussian mutation operator
- Push the newly obtained vector to the population
- Establish the least contributor using the PyGMO.util.hypervolume object
- Remove the least contributor from the population
Script above should produce an output similar to the one below:
P-Distance: 0.77281, Hypervolume: 26999518559.47130
P-Distance: 0.66491, Hypervolume: 26999704053.34119
P-Distance: 0.51330, Hypervolume: 26999704183.90998
.
.
.
P-Distance: 0.00026, Hypervolume: 26999999999.42529
P-Distance: 0.00026, Hypervolume: 26999999999.42529
P-Distance: 0.00024, Hypervolume: 26999999999.42531
We can observe an improvement over the consecutive generations both in the distance to the Pareto front (P-Distance) and the hypervolume indicator itself. The result of the script should be a plot similar to the one below:
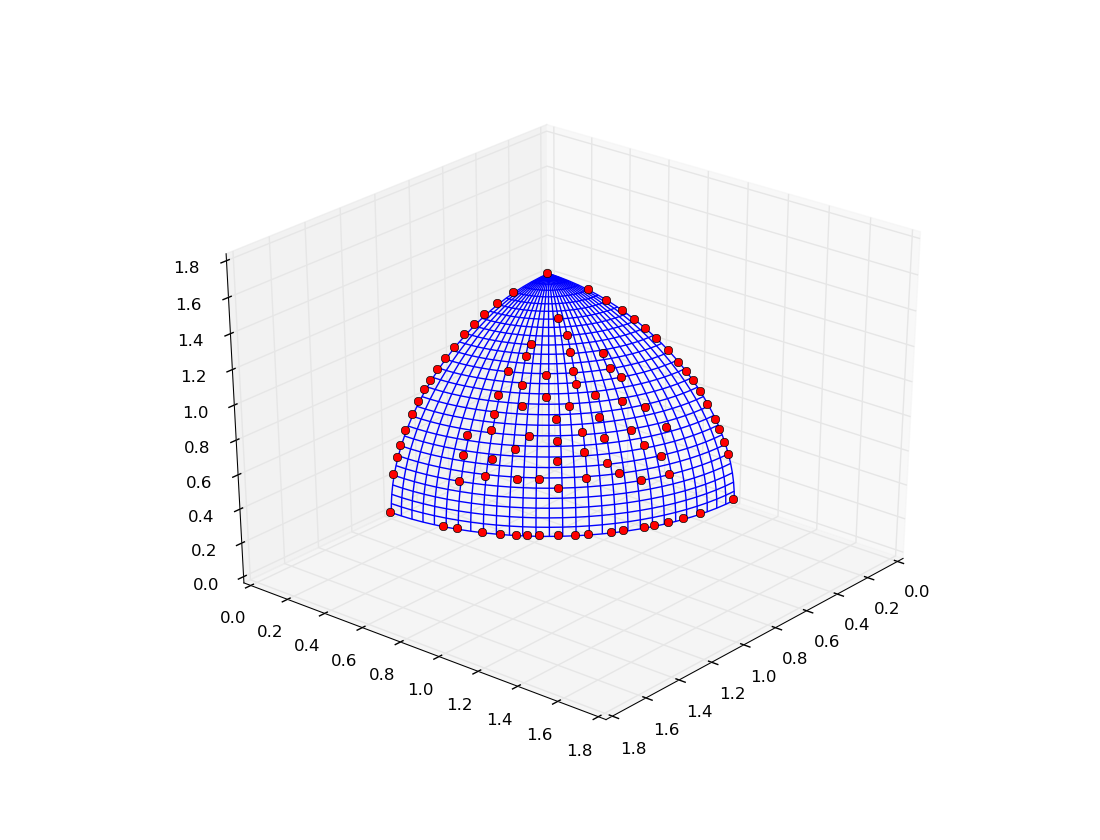
Custom algorithms can also be used in the archipelago evolution. For this, we will employ the information from the previous tutorial Migration based on hypervolume contribution. Substitute the main() method in the script above with the following experiment, which will initiate an archipelago with our custom algorithm on-board.
def main():
prob = problem.dtlz(2)
alg = my_hv_moo_alg(gen = 100, p_m=0.02)
# Initiate the migration policies
s_pol = migration.hv_best_s_policy(0.1, migration.rate_type.fractional)
r_pol = migration.hv_fair_r_policy(0.1, migration.rate_type.fractional)
# Set up the archipelago
n_islands = 16
n_individuals = 64
arch = archipelago(topology=topology.fully_connected())
islands = [island(alg, prob, n_individuals, s_policy=s_pol, r_policy=r_pol) for i in xrange(n_islands)]
for i in islands:
arch.push_back(i)
# Evolve
n_steps = 20
for s in xrange(n_steps):
print "Evolving archipelago, step %d/%d" % (s, n_steps)
arch.evolve(1)
# Merge all populations across the islands together
pop = population(prob)
for isl in arch:
for ind in isl.population:
pop.push_back(ind.cur_x)
print "Final P-Distance: ", prob.p_distance(pop)
prob.plot(pop)
The execution of the script should result in a plot similar to the one below:
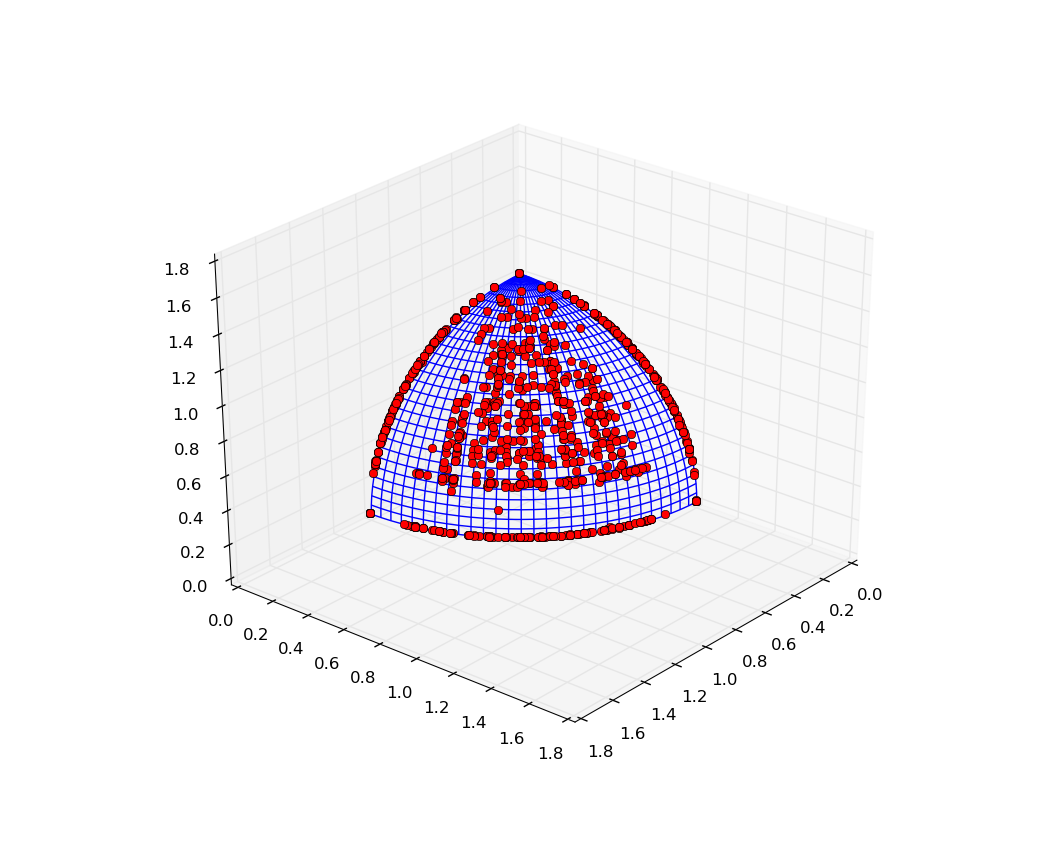