Analysis of the Constraints Landscape¶
In this tutorial we want to show how to use the lanscape anlaysis module Dr. PyGMO for the analysis of the constraint functions.
Instantiate Analysis Module¶
First, we need to instantiate the analysis class as follows:
from PyGMO import *
#We instantiate the optimization problem as usual.
prob=problem.cec2006(5)
#Now we instantiate the analysis class. In this case we will sample 1000 points via via latin
#hypersquare sampling (lhs) and choose output to file.
inspector=util.analysis(prob,1000,'lhs',output_to_file=True)
Tests on constraints functions¶
Now we can start launching the tests related to the constraints functions.
inspector.c_feasibility()
inspector.c_linearity()
inspector.c_regression(degree=[1,1,2,2],interaction=[False,True,False,True])
inspector.c_sensitivity()
The output will look like this:
===============================================================================
ANALYSIS
===============================================================================
-------------------------------------------------------------------------------
PROBLEM PROPERTIES
-------------------------------------------------------------------------------
Problem name: CEC2006 - g5
Global dimension: 4
Integer dimension: 0
Fitness dimension: 1
Constraints dimension: 5
Inequality constraints dimension: 2
Lower bounds: [0, 0, -0.55000000000000004, -0.55000000000000004]
Upper bounds: [1200, 1200, 0.55000000000000004, 0.55000000000000004]
Constraints tolerance: [0.0001, 0.0001, 0.0001, 0, 0]
-------------------------------------------------------------------------------
SAMPLED [1000] POINTS VIA lhs METHOD FOR THE SUBSEQUENT TESTS
-------------------------------------------------------------------------------
C-FEASIBILITY
-------------------------------------------------------------------------------
Constraint h_1 :
Effectiveness >=0 : [0.416]
Effectiveness <=0 : [0.584]
Number of feasible points found : [0]
Constraint h_2 :
Effectiveness >=0 : [0.388]
Effectiveness <=0 : [0.612]
Number of feasible points found : [0]
Constraint h_3 :
Effectiveness >=0 : [0.879]
Effectiveness <=0 : [0.121]
Number of feasible points found : [0]
Constraint g_1 :
Effectiveness >0 : [0.128]
Redundancy wrt. all other ic : [0.0]
Number of feasible points found : [872]
Constraint g_2 :
Effectiveness >0 : [0.124]
Redundancy wrt. all other ic : [0.0]
Number of feasible points found : [876]
Pairwise redundancy (ic) :
_____| g1 | g2 |
g1 | 1.0 | 0.0 |
g2 | 0.0 | 1.0 |
-------------------------------------------------------------------------------
C-LINEARITY
-------------------------------------------------------------------------------
Number of pairs of points used : [1000]
CONSTRAINT PROBABILITY OF LINEARITY
h_1 [0.005]
h_2 [0.005]
h_3 [0.003]
g_1 [1.0]
g_2 [1.0]
-------------------------------------------------------------------------------
C-REGRESSION
-------------------------------------------------------------------------------
CONSTRAINT h_1 :
DEGREE F* R2 R2adj RMSE R2pred PRESS-RMSE
1 126286.466 0.999 0.999 0.006 0.999 0.006
1(i) 126286.466 0.999 0.999 0.006 0.999 0.006
2 348689.605 1.0 1.0 0.002 1.0 0.002
2(i) 348689.605 1.0 1.0 0.002 1.0 0.002
CONSTRAINT h_2 :
DEGREE F* R2 R2adj RMSE R2pred PRESS-RMSE
1 47625.385 0.998 0.998 0.01 0.998 0.01
1(i) 47625.385 0.998 0.998 0.01 0.998 0.01
2 292418.74 1.0 1.0 0.002 1.0 0.002
2(i) 292418.74 1.0 1.0 0.002 1.0 0.002
CONSTRAINT h_3 :
DEGREE F* R2 R2adj RMSE R2pred PRESS-RMSE
1 51587.224 0.998 0.998 0.011 0.998 0.012
1(i) 51587.224 0.998 0.998 0.011 0.998 0.012
2 223152.687 1.0 1.0 0.003 1.0 0.003
2(i) 223152.687 1.0 1.0 0.003 1.0 0.003
CONSTRAINT g_1 :
DEGREE F* R2 R2adj RMSE R2pred PRESS-RMSE
1 2.6e+31 1.0 1.0 1.0e-15 1.0 1.086e-15
1(i) 2.6e+31 1.0 1.0 1.0e-15 1.0 1.086e-15
2 3.907e+30 1.0 1.0 2.581e-15 1.0 2.557e-15
2(i) 3.907e+30 1.0 1.0 2.581e-15 1.0 2.557e-15
CONSTRAINT g_2 :
DEGREE F* R2 R2adj RMSE R2pred PRESS-RMSE
1 1.792e+31 1.0 1.0 1.205e-15 1.0 1.135e-15
1(i) 1.792e+31 1.0 1.0 1.205e-15 1.0 1.135e-15
2 2.322e+30 1.0 1.0 3.347e-15 1.0 3.493e-15
2(i) 2.322e+30 1.0 1.0 3.347e-15 1.0 3.493e-15
-------------------------------------------------------------------------------
C-SENSITIVITY
-------------------------------------------------------------------------------
CONSTRAINT g_1 :
Percentiles : | 0 | 25 | 50 | 75 | 100 |
Gradient norm : | 0.608 | 0.68 | 0.702 | 0.721 | 0.731 |
|dFx|_max/|dFx|_min : | inf | inf | inf | inf | inf |
Gradient sparsity : [0.4]
CONSTRAINT g_2 :
Percentiles : | 0 | 25 | 50 | 75 | 100 |
Gradient norm : | 0.477 | 0.696 | 0.751 | 0.776 | 0.793 |
|dFx|_max/|dFx|_min : | inf | inf | inf | inf | inf |
Gradient sparsity : [0.4]
*Constraints Gradient/Jacobian sparsity plot : <figure_1.png>
*Constraint Gradient/Jacobian PCP plot : <figure_2.png>
*Constraint Gradient/Jacobian PCP plot (inverted) : <figure_3.png>
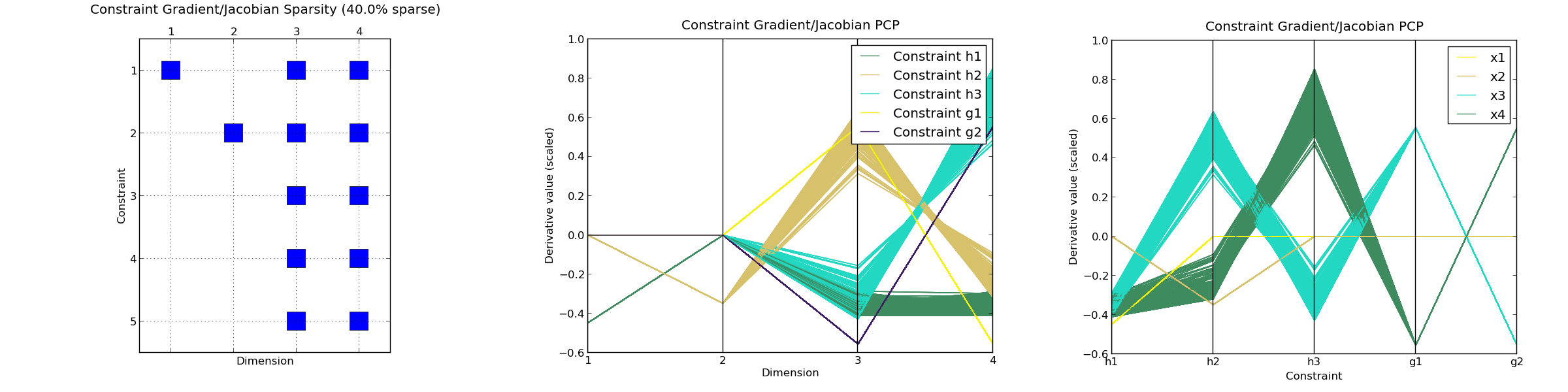